The experts are misinforming us
A recent tweet from Dr. Eric Topol about a study trying to assess the real world effectiveness of Paxlovid provides a really important teachable moment:

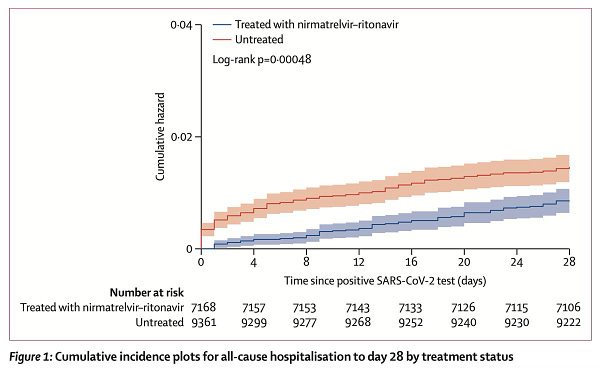
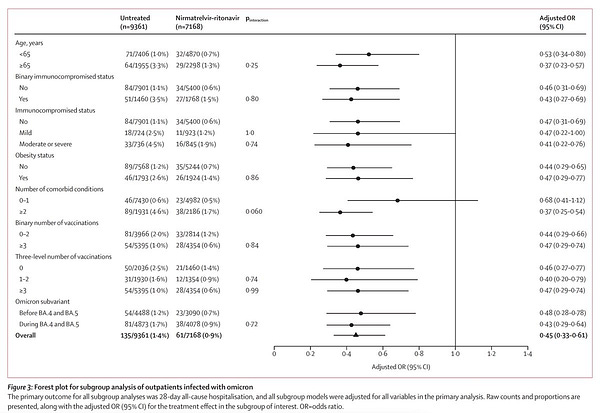
Seem innocuous enough - a study published in the Lancet Infectious Disease that showed a drug that’s being widely used seems to work well.
And keep in mind who this is coming from.
Dr. Topol is one of the closest things that we have in medicine to a mainstream influencer - he has over 600 thousand Twitter followers, he’s regularly quoted in the New York Times, and he’s the author of a bunch of best selling books.
He’s obviously brilliant and influential. And he’s been responsible for uncovering real Pharma wrongdoing - Eric Topol is a giant and has really important accomplishments.
But just because you’re a giant in medicine doesn’t mean that you always interpret evidence correctly.
The study in question is really fishy, and it doesn’t take a lot of digging to see why
Take a closer look at the curves in this study:
Do you see how they’re different from time zero? The Paxlovid group (blue line) has a lower event rate at the beginning of the study than the group that wasn’t treated with Paxlovid (red line).
That suggests a fundamental difference in the composition of the groups that has literally zero to do with Paxlovid treatment.
For this study to be true, you need to believe that Paxlovid works before you start using it.
Immortal time bias is an eternal problem with studies like this, and people were on it from the beginning:

But when confronted with the fact that suggesting this study proves anything is a total overreach, Dr. Topol didn’t back off or admit that perhaps he had overstated the conclusions.
No, he just had a snarky reply to those who questioned the fact that the curves separated too early:

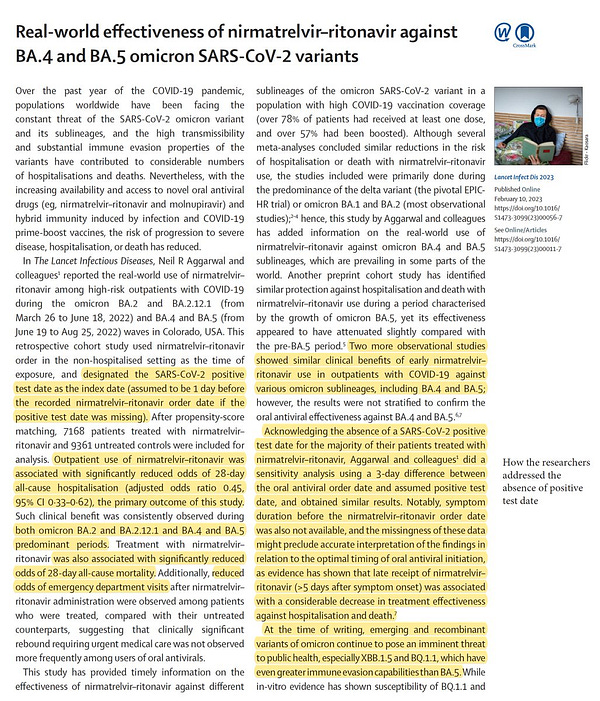
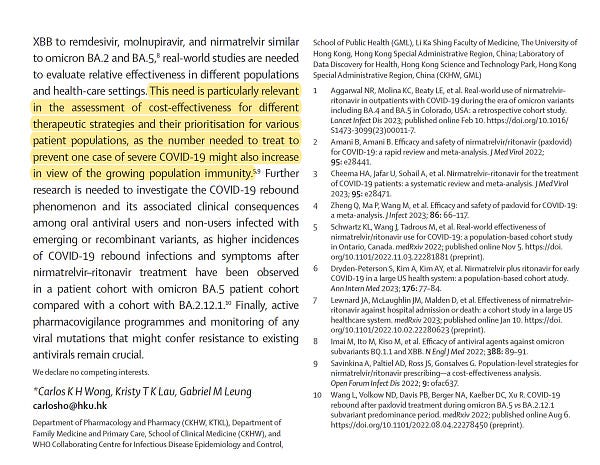
You can point out a number of different problems with this study, but they all kind of miss the point
You can spend a lot of time looking at a trial like this and critiquing the specifics of how we could tell the groups were different in ways that were unrelated to Paxlovid treatment.
The curves separated too early.
They had similar rates of need for extra oxygen and ventilation support, so survival benefit shouldn’t be explained by Covid protection from Paxlovid.
And you can also discuss the protocol of how they counted patients who were hospitalized on the first day of the study, or how they amended the study protocol.
I’m not suggesting that these critiques aren’t important - they certainly are.
But here’s the problem: even if the authors did perfectly account for every single one of these issues, you still can’t draw any conclusions from this trial.
It’s an observational trial. It doesn’t test cause and effect. It’s simply not possible to attribute cause to the intervention here.
The problem isn’t just with the known confounders - it’s with the unknown ones
The only way to determine whether an intervention causes a better outcome is to ensure that the only difference between the group that gets a treatment and the group that doesn’t is the treatment itself.
And so when you hear about a trial like this (which just observed people who got Paxlovid versus those who didn’t), it’s natural to think about what the difference between the two groups might be.
For instance, you can ask about the factors that are associated with access to Paxlovid early in the course of Covid.
You’d probably come up with things like a good relationship with a primary care doctor, high levels of health literacy, having health insurance, socioeconomic status, access to a Covid test and the means to take one, and trust in the health care system.
All things that might also lead to a better Covid outcome, which confound the results.
These confounding variables are really important, but theoretically if you think of them you can perform analytics to adjust for these things.
The problem with observational studies is that there are always going to be differences that you can’t account for.
We call these “unmeasured confounders.” Donald Rumsfeld would have called them unknown unknowns.
That’s the magic of a randomized trial - it allows you manipulate the variable of interest to study causation and reduce confounding.
I don’t understand why a study like this is even done, let alone published
I’m not being snarky with that header - I literally do not understand why these studies are done.
A study like this adds nothing to our understanding of who should get Paxlovid and who shouldn’t.
It doesn’t help with figuring out the effect size of a benefit so you can perform a cost/benefit analysis.
It doesn’t even confirm whether Paxlovid works in people who have previously been vaccinated for Covid.
And so just because an observational trial like this can be done, I would argue that it really shouldn’t be done, because it just obfuscates rather than informs.
The people who performed this study are obviously smart and statistically savvy - but their efforts are wasted on a study that’s directly designed to misinform us.
When the experts of the world don’t interpret data correctly, we all suffer
Eric Topol has the type of platform that I will never have. When he speaks, a lot of incredibly powerful people listen. His words influence policy.
But he knows better than this. Or at least he should.
An observational trial does not prove cause and effect because it is designed in a way that it simply cannot prove it.
When someone with his megaphone uses it to express misinformation, he undermines trust in the scientific data.
And that’s what this is - it’s misinformation.
You’ve probably heard a lot about the concept of “misinformation” when it comes to the pandemic. Usually the word is thrown about for people who are incorrectly talking about the dangers of vaccination, the benefits of ivermectin, or the lack of harms that Covid has had.
But I would argue that what Eric Topol is doing with a tweet like this is also spreading misinformation - and just because I agree with a lot of his policy views, that doesn’t mean I am going to cheerlead while he overstates the conclusions of this type of work.
It’s disappointing how much commentary is made on studies like this, studies that I believe are literally pointless to do.
And it’s really disconcerting how much policy is made based on results like this - and how much people who have big platforms (even the ones who I may agree with politically) use them to spread misinformation.